An Introduction to AI Chatbots
In recent years chatbots have become an integral part of companies’ customer service offering, providing benefits such as:
- Operational efficiency – the capacity to resolve mass queries at scale
- Improved CSAT scores – utilising Natural Language Processing (NLP) for the best customer experience
- Instant, 24/7 support – providing customers with around the clock assurance
- Seamless customer journeys – guiding customers to the intended result
- Brand personification – chatbots can be customised to represent company character
- Lead generation – automating the qualification process for efficiency
- Enhanced CX – Machine Learning principles enable improved experiences
- Improved employee morale – automating routine queries allows agents to focus on complexities
- Smooth escalation – identifying when a customer should be transferred to a human
So how does it work?
AI-powered chatbots utilise intelligent algorithms, Machine Learning (ML) principles and Natural Language Processing (NLP) to ‘learn’ from user behaviours and patterns to become more effective over time. Usually integrated with a centralised knowledge base, an AI chatbot can interpret query variations to identify the adequate result and deliver it using a conversational manner. The more interactions the chatbot has, the ‘smarter’ it becomes.
It is important to note that there is a key difference between an AI chatbot and a chatbot, one relies on artificial intelligence and the other relies on rule-based automations:
- Basic, rule-based chatbots: built on simple FAQ automations, this type of chatbot can only respond to queries that exactly match its pre-loaded responses. These will only trigger when specific keywords are entered by the user, any variations will not register. The most common answer these chatbots serve is “I don’t understand the question”.
- AI-powered chatbots: using multi-layered algorithmic Natural Language Understanding (NLU), these chatbots understand customer intent and respond conversationally using Natural Language Generation (NLG). This approach will deliver the right response, regardless of how a query is phrased.
Basic, rule-based chatbot
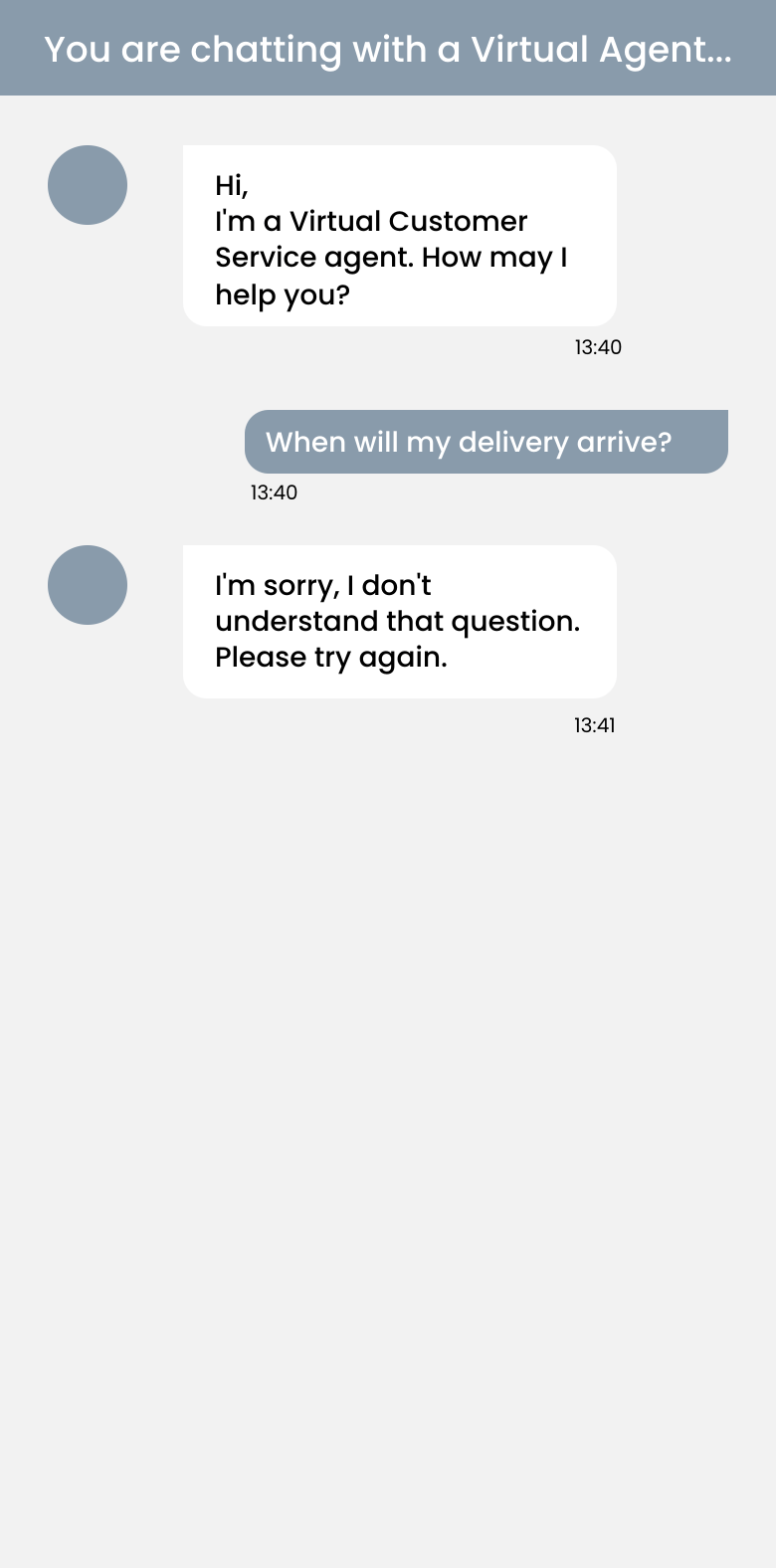
AI-powered chatbot
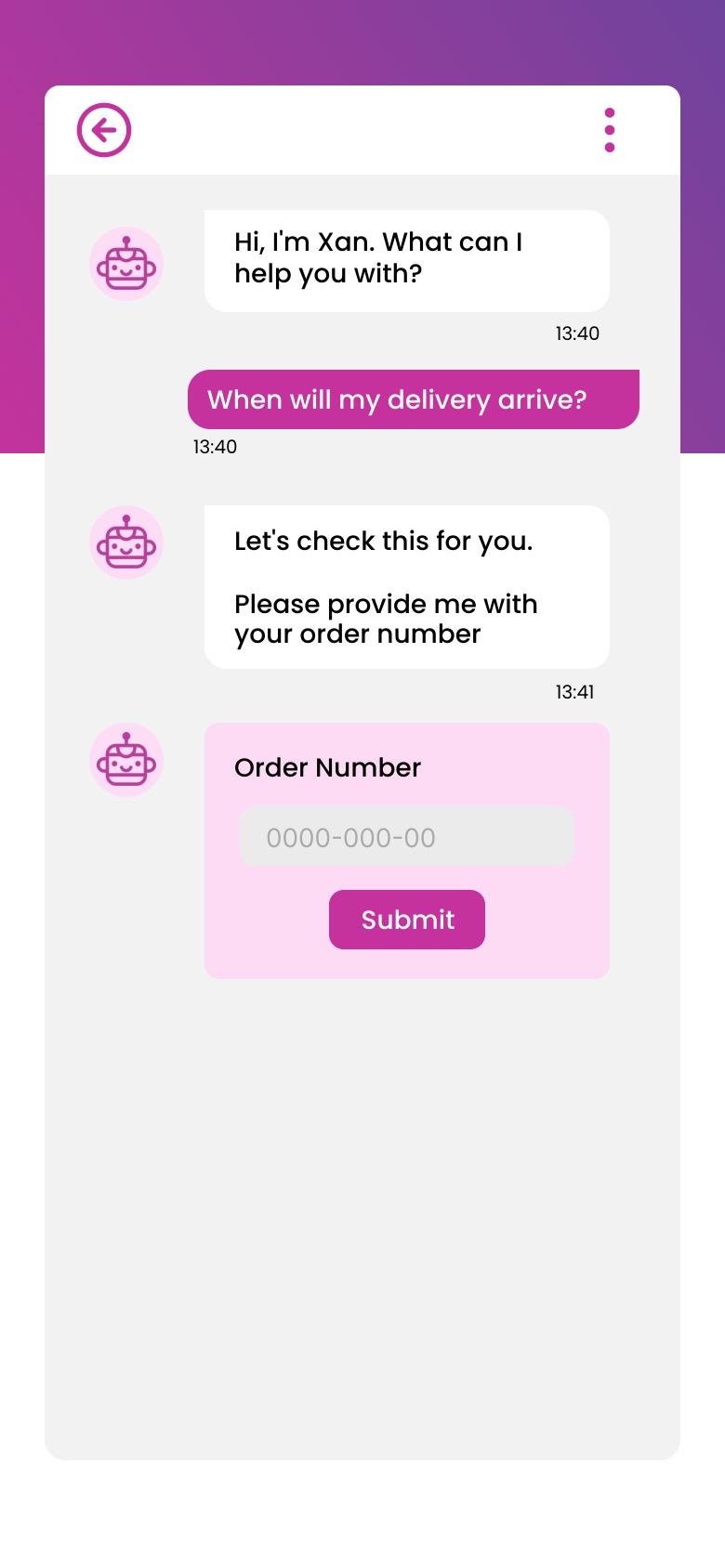
The global chatbot market is expected to grow by $1.11 billion during 2020-2024 – and CAGR of 29%. But how did we get here?
Early AI Chatbots
Artificial intelligence and bots can be traced back to as early as the 1950s when Turing famously designed the Turing Test which would determine whether a machine could pass as a human based on the answers it gave.
But let’s focus on 1990s when the then, “chatterbot” was born. Unlike the chatbots of today, the initial wave of chatterbots weren’t created to facilitate customer service. Instead they were essentially a toy that was played with to test the bot’s intelligence. The way these chatbots learnt was from people talking to them – the more intel their community fed them, the greater their database of ‘knowledge’ became.
Cleverbot (1997) and other chatterbots constructed their replies by drawing on their database of previous conversations rather than consulting a regulated set of knowledge articles through NLP. This quickly became problematic when it was made available to the public.
Why?
- Because people were intentionally ‘teaching’ the chatbots offensive ‘knowledge’, it would serve users just that.
- Due to the nature of the learning process, there was no control over the content that was being delivered, this meant no way to prevent profanity or inappropriate ‘views’ being shared by the bots.
- These chatbots were viewed simply as toys, they learnt nothing of true value and didn’t serve a purpose other than to entertain.
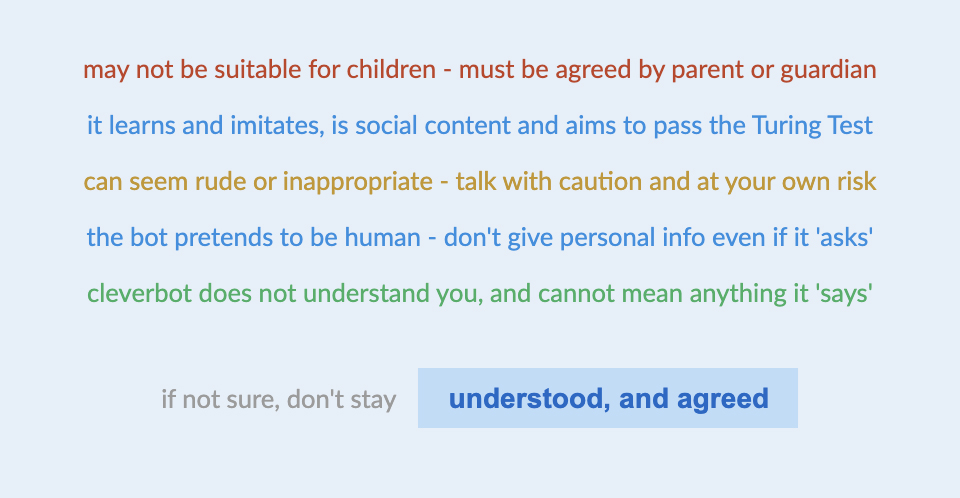
Following this, there were several developments to try and introduce Machine Learning (ML) chatbots, including Microsoft’s Tay AI (2016). This wasn’t specifically focused on customer service like the chatbots of today but was Microsoft’s attempt to find out if a chatbot could learn like a human.
Tay AI was launched on twitter as a novelty bot, using the tweets she was sent as her training data, Microsoft explained: “The more you chat with Tay the smarter she gets.” The fundamental issue here was the uncontrolled training data – Tay was learning from users who were tweeting their offensive, inflammatory views and once she has drawn similarities and identified patterns, she began to repeat such statements. Her sentence structures were sound, but their content was nonsensical and often controversial.
This is an example of how unregulated training data doesn’t work for customer service chatbots, whilst she managed to learn punctuation and how to form a sentence, she could not identify that what she was saying was offensive. Tay AI backfired for Microsoft and was removed from Twitter after just 16 hours.
Modern Day AI Chatbots
Today, AI chatbots don’t use unregulated or generalised training data, instead they utilise carefully curated data based on customer needs. Such data is usually stored in a centralised knowledge base which is configured to contain ML within set parameters so that companies can reap the benefits of using a chatbot, whilst avoiding fiascos such as Tay AI’s.
Modern day AI chatbots are most commonly used in customer service and use NLP to deliver results in a conversational way. Once a user enters their query, intelligent algorithms and NLP analyse the query, dissecting the sentence into search terms, grammar, and keyword popularity to serve relevant articles to the user.
If there is not an obvious match available then some AI-powered chatbots use additional search layers such as Synthetix’s “Jabberwocky”, which utilises NLP to understand sentence structure. It carefully unpicks the sentence into word classes and identifies conversational responses based on the proprietary Natural Language Generation (NLG) which can also be manually edited and configured by the Knowledge Manager.
The purpose of such additional search layers is to inject brand personality into the conversation whilst improving the accuracy of answers provided. It also ensures that customers will always receive a conversational response, not “I’m sorry, I don’t understand that question” – which proves frustrating for customers, thus poor CX.
The AI-driven chatbots of today are significantly more controlled than those of recent decades with greater efficiency of customer service but with the same, engaging human-like interactions.
AI Chatbots In Customer Service
AI chatbots are considered a crucial part of a company’s online customer service offering and can prove invaluable to both customers and teams across a multitude of industries.
AI Chatbots For Customers
When executed well, chatbots are considered a valuable tool for customers, providing them with quick answers 24/7 through a convenient platform. This is especially useful for those in emergency, out of hours situations who require real-time access to contact details for assistance, or those who simply don’t have time to engage with agent-assisted channels.
AI chatbots can significantly improve customer experience through customer journey technology and ML principles. Certain chatbot solutions utilise decision tree technology to effectively guide the customer to their intended destination. Whether that be a knowledge article, product page or social channel, decision trees, that are carefully configured by a Knowledge Manager, ask a set of questions that will ultimately determine the customer journey and end goal. Without such measures in place, the chatbot assumes that the customer has carried out all necessary problem solving themselves – which is rarely true. The same technology can identify when it is best for an agent to intervene and can seamlessly escalate a customer to an agent assisted channel such as live chat.
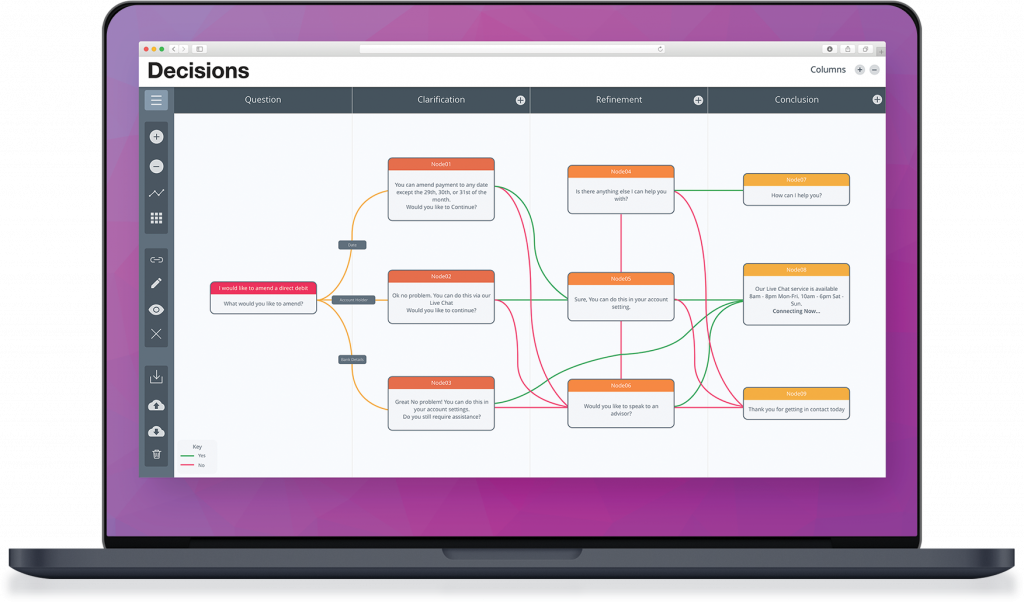
Moreover, chatbots can learn from every customer interaction, storing popular keywords, grammar and colloquialisms for future conversations – constantly improving CX.
Read more about customer service chatbots here.
AI Chatbots For Teams
Customer service and contact centre teams can benefit significantly from introducing chatbots into their offering. Because they are powered by AI and configured to utilse NLP, the accuracy, efficiency and quality of answers delivered is of a high standard, this improves over time as bots learn from their interactions, having a positive effect on CSAT scores.
Chatbots allow routine questions to be automated, this results in mass queries being resolved at scale promoting not only operational efficiency but reducing overheads. Accumulated operational and staffing costs are cut because a large portion of queries are dealt with digitally and via a bot. Additionally, because routine queries are automated, contact centre employees are given greater bandwidth to deal with more complex, sensitive and subjective issues. This encourages job satisfaction, empowers employees and impacts positively on staff attrition rates.
Chatbots can also directly attribute to revenue streams. Acting as a lead generation tool, some chatbots are configured to qualify leads before they are passed on to sales teams. Equipped with data including the questions they have asked, the preferences they have chosen and using data capture functions, their contact details, leads can be instantly escalated to an agent to nurture. This is particularly useful for teams who previously would have no intelligence on potential ‘hot leads’, but through NLP, can be identified and moved into the sales funnel.
If you enjoyed this article and would like to know more about AI chatbots, you can read more here or